Artificial Intelligence
AI-Driven Data Analysis: The Future of Big Data
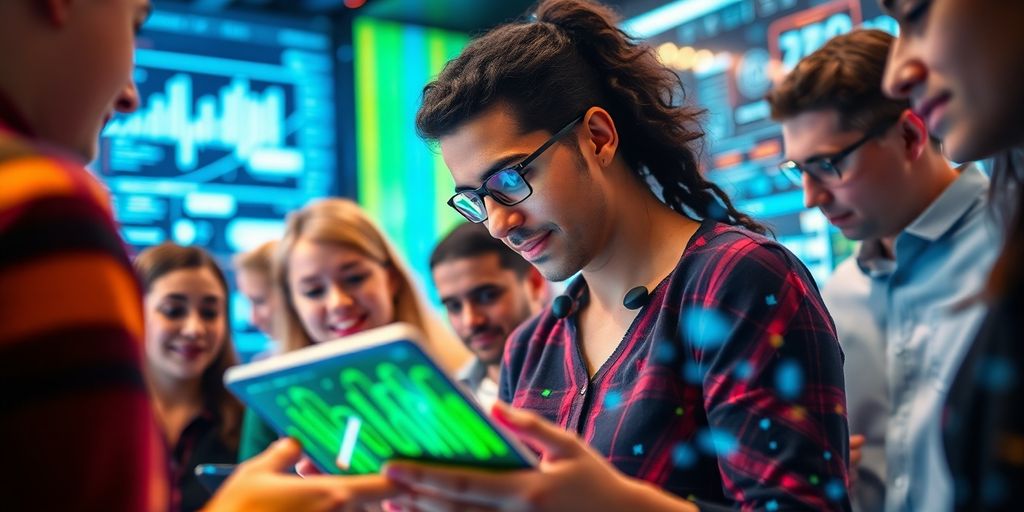
Artificial Intelligence (AI) is changing the way we analyse data, making it faster and smarter. This article explores how AI is shaping the future of big data, helping businesses make better decisions, understand their customers, and solve complex problems in various fields like healthcare and finance. We will also discuss the challenges and ethical considerations that come with these advancements.
Key Takeaways
- AI helps predict future trends by analysing past data, improving decision-making.
- Real-time data analysis allows businesses to react quickly to changes and challenges.
- Personalised services driven by AI enhance customer satisfaction and loyalty.
- AI is transforming healthcare by improving diagnostics and predicting patient outcomes.
- Ethical use of AI is crucial to ensure data security and responsible practises.
Enhanced Decision Making with Predictive Analytics
Understanding Predictive Analytics
Predictive analytics uses historical data to forecast future events. By identifying patterns, businesses can make smarter choices. This method is crucial for understanding customer behaviour and market trends.
Applications in Various Sectors
Predictive analytics is used in many fields, including:
- Retail: Anticipating customer demand to manage inventory.
- Finance: Assessing risks and predicting market movements.
- Healthcare: Forecasting patient outcomes and optimising treatments.
Benefits of Predictive Analytics
The advantages of using predictive analytics include:
- Informed Decision-Making: Helps businesses make data-driven choices.
- Cost Efficiency: Reduces waste by predicting needs accurately.
- Competitive Advantage: Enables companies to stay ahead of market trends.
Predictive analytics transforms data into actionable insights, allowing businesses to adapt and thrive in a fast-paced environment.
Sector | Key Benefit | Example Use Case |
---|---|---|
Retail | Demand forecasting | Stock management |
Finance | Risk assessment | Investment strategies |
Healthcare | Patient outcome prediction | Treatment planning |
Real-Time Analytics for Immediate Insights
Importance of Real-Time Data
Real-time data is crucial for businesses that need to make quick decisions. Having access to live data allows companies to respond to changes in the market or customer behaviour instantly. This can lead to better outcomes and improved efficiency.
Technologies Enabling Real-Time Analytics
Several technologies make real-time analytics possible:
- Stream Processing: This technology allows data to be processed as it arrives, rather than waiting for batches.
- In-Memory Computing: This speeds up data processing by storing data in RAM instead of on slower disc drives.
- Cloud Computing: Cloud services provide scalable resources that can handle large volumes of data quickly.
Use Cases of Real-Time Analytics
Real-time analytics can be applied in various fields:
- Retail: Stores can track inventory levels and customer purchases to optimise stock.
- Healthcare: Hospitals can monitor patient vitals in real-time, improving response times.
- Finance: Banks can detect fraudulent transactions as they happen, protecting customers.
Real-time analytics is transforming how businesses operate, enabling them to make informed decisions faster than ever before.
In summary, real-time analytics is essential for businesses aiming to stay competitive in a fast-paced environment. By leveraging the right technologies, organisations can gain immediate insights that drive better decision-making and enhance overall performance.
Customised User Experiences Through Personalisation
Role of AI in Personalisation
AI plays a crucial role in creating tailored experiences for customers. By analysing individual data, AI can predict what customers might like or need. This means businesses can offer products and services that are more relevant to each person.
Impact on Customer Satisfaction
When customers receive personalised experiences, their satisfaction often increases. Here are some key benefits:
- Increased loyalty: Customers are more likely to return if they feel understood.
- Better engagement: Personalised messages grab attention more effectively.
- Higher conversion rates: Targeted promotions lead to more sales.
Examples of Personalised Services
Many companies are using AI to enhance their services. Here are a few examples:
- Streaming services: They recommend shows based on viewing history.
- E-commerce sites: They suggest products based on past purchases.
- Email marketing: Businesses send tailored offers to specific customer segments.
Personalisation is not just a trend; it’s becoming a necessity in today’s market. Companies that embrace it can stand out and build stronger relationships with their customers.
Service Type | Personalisation Method | Example |
---|---|---|
Streaming Services | Viewing history analysis | Netflix recommendations |
E-commerce | Purchase history analysis | Amazon product suggestions |
Email Marketing | Customer segmentation | Targeted promotional emails |
Driving Innovation in Healthcare
AI in Medical Diagnostics
AI is changing the way we diagnose diseases. By analysing large amounts of medical data, AI can help doctors identify illnesses more accurately and quickly. This leads to better patient outcomes. For example, AI can process images from X-rays or MRIs to spot issues that might be missed by the human eye.
Predicting Patient Outcomes
AI can also predict how patients will respond to treatments. By looking at past data, AI can help doctors choose the best treatment plans for individual patients. This means that patients can receive care that is tailored to their specific needs, improving their chances of recovery.
Data-Driven Medical Research
AI is revolutionising medical research by analysing vast datasets to find new treatments and understand diseases better. Researchers can use AI to:
- Identify patterns in patient data
- Discover new drug candidates
- Accelerate clinical trials
In the world of healthcare, using AI to analyse data is not just about efficiency; it’s about saving lives and improving the quality of care.
By integrating AI into healthcare, we are not only enhancing diagnostics and treatment but also paving the way for a future where patient care is more personalised and effective. This innovation is crucial for tackling the challenges faced by the healthcare industry today.
Tackling Big Data Challenges with AI
Big data presents several challenges, including data quality, storage, and security. AI technologies are stepping in to help solve these issues, making data not just large but also valuable and manageable.
Data Quality and AI
AI can significantly improve data quality by:
- Identifying errors and inconsistencies in datasets.
- Automating data cleaning processes to ensure accuracy.
- Learning from user interactions to detect common mistakes.
AI for Data Storage Solutions
AI can optimise data storage by:
- Predicting storage needs based on usage patterns.
- Automating data archiving processes to free up space.
- Enhancing data retrieval speeds through intelligent indexing.
Ensuring Data Security with AI
AI plays a crucial role in data security by:
- Monitoring systems for unusual activities.
- Identifying potential threats in real-time.
- Automating responses to security breaches to minimise damage.
In the world of big data, AI is not just a tool; it’s a game changer. It helps organisations turn challenges into opportunities, ensuring that data remains a powerful asset rather than a burden.
Ethical Considerations and Responsible Use
As we explore the potential of AI and big data, it is crucial to think about the ethical implications. Here are some key points to consider:
Ethical Implications of AI
- Privacy: Protecting personal information is essential.
- Bias: AI systems can reflect biases present in their training data.
- Transparency: Understanding how AI makes decisions is important.
Ensuring Responsible AI Use
- Data Protection: Implement strong security measures to safeguard data.
- Fairness: Strive for fairness in AI algorithms to avoid discrimination.
- Accountability: Establish clear guidelines for AI usage and decision-making.
Regulations and Guidelines
Regulation | Description |
---|---|
GDPR | Protects personal data in the EU. |
CCPA | Enhances privacy rights for California residents. |
AI Ethics Guidelines | Frameworks for ethical AI development and use. |
The future of AI and big data must be built on a foundation of trust, ensuring that technology serves humanity responsibly.
By addressing these ethical considerations, we can harness the power of AI while ensuring it is used responsibly and ethically.
Synergy Between AI and Big Data
The relationship between AI and big data is truly powerful. They work together to enhance data analysis and decision-making processes. Here’s how they connect:
How AI Enhances Big Data
- Data Processing: AI helps in processing large datasets quickly, making it easier to find patterns and insights.
- Predictive Analytics: By analysing historical data, AI can predict future trends, which is crucial for businesses.
- Automation: AI automates many tasks, reducing the time and effort needed for data analysis.
Big Data as Fuel for AI
- Learning Source: AI needs vast amounts of data to learn and improve its algorithms.
- Improved Accuracy: The more data AI has, the better it can make decisions and predictions.
- Real-Time Insights: Big data allows AI to provide insights almost instantly, which is vital in fast-paced environments.
Technological Innovations from Synergy
- Enhanced Tools: The combination of AI and big data leads to the development of advanced analytical tools.
- New Applications: This synergy creates opportunities in various fields, from healthcare to finance.
- Data-Driven Decisions: Businesses can make informed decisions based on accurate data analysis.
The collaboration between AI and big data is reshaping how we understand and use information, leading to smarter decisions and innovative solutions.
In summary, the synergy between AI and big data is not just beneficial; it is essential for modern data analysis. By leveraging both, organisations can unlock new insights and drive significant improvements in their operations.
AI-Powered Data Analytics vs. Traditional Analytics
Differences Between AI and Traditional Analytics
AI-powered analytics and traditional analytics differ significantly in their approach and capabilities. Here are some key differences:
- Automation: AI can automate data processing, reducing human error and speeding up analysis.
- Real-Time Insights: AI provides immediate insights, while traditional methods often involve delays.
- Complexity Handling: AI can manage vast amounts of data and uncover hidden patterns, unlike traditional analytics.
Advantages of AI-Powered Analytics
The benefits of using AI in data analytics are substantial:
- Speed: AI processes data much faster than traditional methods.
- Accuracy: AI reduces human error, leading to more reliable insights.
- Predictive Capabilities: AI can forecast future trends based on historical data, which traditional analytics cannot do effectively.
Feature | AI-Powered Analytics | Traditional Analytics |
---|---|---|
Data Processing Speed | High | Moderate |
Real-Time Analysis | Yes | No |
Predictive Insights | Yes | Limited |
Automation of Tasks | Yes | No |
Limitations of Traditional Analytics
While traditional analytics has its place, it comes with limitations:
- Static Reports: Traditional analytics often produces fixed reports that do not adapt to new data.
- Limited Predictive Power: It struggles to provide actionable insights for future events.
- Manual Data Handling: Requires significant human intervention, increasing the risk of errors.
In the evolving landscape of data analysis, AI is transforming how businesses operate, offering unprecedented insights and efficiency.
By understanding these differences, organisations can better leverage the strengths of AI-powered analytics to enhance their decision-making processes and drive growth.
Implementing AI Analytics in Organisations
Steps for Successful Implementation
Implementing AI analytics in your organisation can be straightforward if you follow these key steps:
- Define Your Goals: Start by understanding what you want to achieve with AI analytics. This could be improving customer service or increasing sales.
- Engage Stakeholders: Get support from everyone involved. It’s important that all team members understand the value of AI analytics and are willing to learn.
- Assess Resources: Determine what tools and skills you need. This includes software, hardware, and training for your team.
Challenges and Solutions
While implementing AI analytics, you may face some challenges:
- Data Quality: Ensure your data is accurate and reliable. Poor data can lead to incorrect insights.
- Integration Issues: Make sure your AI tools can work with your existing systems. This may require additional software or adjustments.
- Resistance to Change: Some team members may be hesitant to adopt new technologies. Provide training and support to ease this transition.
Getting Stakeholder Buy-In
To successfully implement AI analytics, it’s crucial to gain support from all levels of your organisation. Here are some tips:
- Communicate Benefits: Clearly explain how AI analytics can improve processes and outcomes.
- Involve Key Players: Include influential team members in the planning process to foster a sense of ownership.
- Provide Training: Offer training sessions to help everyone understand how to use the new tools effectively.
AI analytics can transform your organisation by providing valuable insights that drive better decision-making.
By following these steps, you can effectively implement AI analytics and harness its power to enhance your organisation’s performance.
In summary, implementing AI analytics involves defining clear goals, engaging stakeholders, assessing resources, and overcoming challenges. With the right approach, your organisation can leverage AI to gain insights and improve decision-making.
Future Trends in AI-Driven Data Analysis
Emerging Technologies
The landscape of data analysis is rapidly changing, driven by new technologies. Some key advancements include:
- Machine Learning: Enhances data processing and predictive capabilities.
- Natural Language Processing: Allows for better interaction with data through human language.
- Cloud Computing: Facilitates scalable data storage and analysis.
Predicted Market Growth
The global data analytics market is set to grow significantly. Projections indicate:
- 2023: $61.44 billion
- 2033: $581.34 billion
This growth reflects the increasing importance of data in decision-making across industries.
Future Use Cases
As AI continues to evolve, we can expect to see:
- Automated Data Cleaning: Reducing the time analysts spend on preparing data.
- Enhanced Personalisation: Tailoring services to individual customer needs.
- AI-Driven Insights: Providing deeper understanding of complex data sets.
The future of data analysis lies in the collaboration between AI and human analysts, where each complements the other’s strengths.
In summary, the future of AI-driven data analysis is bright, with substantial investments and innovations paving the way for more efficient and insightful data practises.
The Human Edge in the Age of AI
Role of Human Analysts
In a world increasingly dominated by AI, human analysts remain essential. They bring unique skills that machines cannot replicate, such as creativity, emotional intelligence, and critical thinking. While AI can handle repetitive tasks and process vast amounts of data, it is the human touch that adds value to data interpretation and decision-making.
Complementing AI with Human Insight
Analysts will not be replaced; instead, they will evolve into navigators of data. Their role will shift from merely processing information to telling compelling stories with data. This transformation requires:
- Strong communication skills to convey insights effectively.
- Collaboration with AI systems to enhance decision-making.
- Continuous learning to keep up with technological advancements.
Future of Data Science Careers
As AI continues to grow, new job roles will emerge, focusing on the synergy between human skills and AI capabilities. Some potential roles include:
- AI trainers who teach machines to understand human nuances.
- Data ethicists who ensure responsible AI use.
- AI operations specialists who manage AI systems in organisations.
The future of data analysis is not about replacing humans but enhancing their capabilities with AI. This partnership will drive innovation and growth in the data-driven economy.
In conclusion, while AI will change the landscape of data analysis, the human edge will always be vital. Analysts will thrive by leveraging their unique skills alongside AI, ensuring that data-driven decisions are not only informed but also insightful and ethical.
Conclusion
In conclusion, the combination of AI and big data is set to change how we understand and use information. With AI’s ability to analyse large amounts of data quickly, businesses can make smarter choices and respond to changes in real time. This not only helps in improving customer experiences but also drives innovation in various fields like healthcare. However, as we embrace these technologies, we must also think about the ethical implications and ensure that we use AI responsibly. The future of data analysis looks bright, and with careful consideration, we can harness its full potential.
Frequently Asked Questions
What is predictive analytics and how does it help in decision-making?
Predictive analytics uses past data to forecast future events. It helps businesses make smarter choices by spotting trends and patterns.
Why is real-time analytics important?
Real-time analytics allows businesses to react quickly to changes. This is vital in areas like finance and customer service where timing is crucial.
How does AI improve personalisation for users?
AI helps businesses understand what each customer likes. This way, they can offer tailored services, making customers happier.
In what ways is AI transforming healthcare?
AI is changing healthcare by helping doctors diagnose illnesses faster, predict patient outcomes, and find new treatments through research.
What challenges does big data face, and how can AI help?
Big data can be messy and hard to manage. AI can improve data quality, storage solutions, and keep information secure.
What are the ethical concerns surrounding AI?
Using AI raises questions about privacy and fairness. It’s important to ensure that AI is used responsibly and follows guidelines.
How do AI and big data work together?
AI needs lots of data to learn and improve. Big data provides this information, while AI helps make sense of it quickly.
What future trends can we expect in AI-driven data analysis?
We can expect new technologies to emerge, more businesses to adopt AI, and innovative uses of data to grow in the coming years.
-
Religion & Spirituality5 days ago
Faith Meets Science: Christianity in the 21st Century
-
Education5 days ago
A Visionary in Screen Acting Education
-
Legal News4 days ago
From Denial to Victory: MTMC’s Proven Strategies for Tort Appeal Success
-
Business3 days ago
Raise the Bar and Expect the Best
-
Lifestyle5 days ago
Bridezillas and Toddlers Are Pretty Similar
-
Religion & Spirituality5 days ago
Church in Action: Faith’s Answer to Social Justice Starts in the Streets
-
Healthcare3 days ago
Florida Faces a Silver Tsunami as Aging Boomers Flock to the Sunshine State
-
Press Release4 days ago
Orion Depp Reissues Pioneering Binance (BNB) Crypto Investment Thesis from 2018