Artificial Intelligence
Artificial Intelligence Ethics: Balancing Innovation with Responsibility
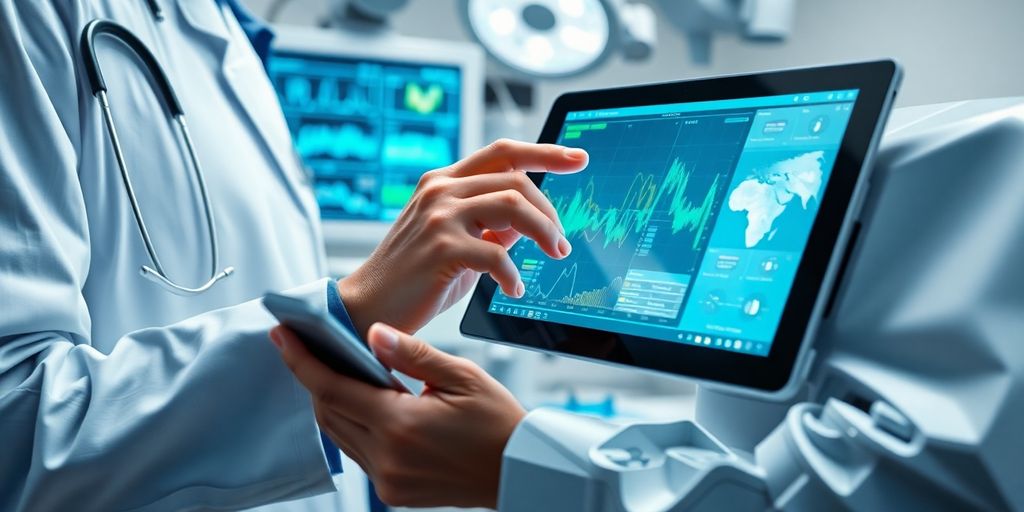
As artificial intelligence (AI) continues to evolve, its ethical implications become increasingly significant. This article explores the delicate balance between innovation and responsibility, highlighting the core principles that should guide AI development. By focusing on fairness, accountability, and transparency, we can ensure that AI serves humanity positively without causing harm.
Key Takeaways
- AI must be fair and non-discriminatory, ensuring everyone is treated equally.
- Transparency is crucial; people should understand how AI makes decisions.
- Accountability is essential; developers must take responsibility for AI outcomes.
- Data privacy must be safeguarded to protect individuals’ rights.
- Human involvement is necessary to oversee AI systems and maintain ethical standards.
Core Principles of Artificial Intelligence Ethics
Fairness and Non-Discrimination
AI systems must be designed to avoid bias and ensure that all individuals are treated equally. This means that developers should actively work to prevent any form of discrimination in their algorithms.
- Key Actions:
- Use diverse datasets for training.
- Regularly test AI systems for bias.
- Implement fairness metrics to evaluate outcomes.
Transparency and Explainability
It is crucial for AI systems to be transparent, allowing users to understand how decisions are made. This builds trust and accountability.
- Key Actions:
- Provide clear documentation of AI processes.
- Ensure that users can access explanations for decisions.
- Engage in open discussions about AI functionalities.
Accountability and Responsibility
Establishing clear accountability is essential for the ethical use of AI. Developers and users must be responsible for the outcomes of AI systems.
- Key Actions:
- Define roles and responsibilities in AI projects.
- Create frameworks for addressing negative impacts.
- Encourage a culture of ethical responsibility among AI practitioners.
In summary, the core principles of AI ethics focus on fairness, transparency, and accountability, ensuring that AI technologies benefit society while minimising harm.
These principles guide the development and deployment of AI, helping to create systems that are not only innovative but also responsible and ethical.
Highlight: The importance of fairness and accountability cannot be overstated, as they are fundamental to building trust in AI technologies.
The Role of Human Oversight in AI Systems
Importance of Human Involvement
Human oversight is crucial in the development and operation of AI systems. AI can automate many tasks, but it is essential for humans to be involved at every stage. This ensures that the systems are functioning correctly and that their outputs are reliable. Without human involvement, AI can lead to unintended consequences, as seen in various incidents where AI systems behaved unexpectedly.
Balancing Automation with Human Control
To maintain a balance between automation and human control, organisations should:
- Implement regular audits of AI systems to ensure they meet ethical standards.
- Establish clear lines of responsibility for AI decisions and actions.
- Incorporate diverse perspectives in AI development teams to address potential biases.
Case Studies of Human Oversight
Several case studies highlight the importance of human oversight in AI:
- Bing’s chatbot incident: This case demonstrated how AI can mimic harmful behaviours if not properly monitored.
- Self-driving car accidents: These incidents underline the need for human intervention in critical situations.
- Microsoft’s transparency reports: Regular audits and transparency measures have helped build trust in AI systems.
Involving humans in AI processes not only enhances the reliability of the systems but also fosters public trust in their use.
By ensuring that humans are an integral part of AI systems, we can better navigate the challenges and responsibilities that come with this technology. This approach is vital for achieving a balance between innovation and ethical responsibility, ultimately leading to a more trustworthy AI landscape.
Ensuring Data Privacy and Security in AI
In the age of AI, protecting personal information is more important than ever. As AI systems rely heavily on data, ensuring privacy and security is crucial for maintaining trust.
Data Protection Protocols
- Informed Consent: Users should be aware of how their data is collected and used.
- Data Anonymization: Removing personal identifiers helps protect individual identities.
- Access Controls: Limiting who can access sensitive data is essential for security.
Privacy-Enhancing Technologies
AI can be used to improve data security. For example, systems like Darktrace monitor network traffic to detect unusual activities that may indicate a breach. This proactive approach can significantly enhance personal data protection.
Contingency Planning for Data Breaches
- Develop a Response Plan: Outline steps to take in case of a data breach.
- Regular Security Audits: Conduct audits to identify vulnerabilities.
- User Notification: Inform affected users promptly to mitigate risks.
Protecting data privacy and security is not just a legal obligation; it is a moral responsibility that builds trust between users and AI systems.
By implementing these strategies, organisations can ensure that they are safeguarding sensitive information while harnessing the power of AI.
Ethical AI Design and Development
Integrating Ethics into AI Design
Incorporating ethical considerations into AI design is essential to avoid negative outcomes. This means that developers should think about the impact of their systems on society. Here are some key points to consider:
- User Impact: How will the AI affect users and their privacy?
- Bias Prevention: What steps are taken to avoid bias in AI decisions?
- Transparency: Are the AI’s processes clear and understandable?
Multidisciplinary Governance Frameworks
Creating a team that includes various experts can help ensure ethical AI development. This team might include:
- Ethicists
- Sociologists
- Technologists
This diverse approach helps to prioritise ethical issues and create a balanced perspective.
Challenges in Ethical AI Implementation
Implementing ethical AI can be tough. Some challenges include:
- Resistance to Change: Teams may be reluctant to adopt new ethical practises.
- Lack of Resources: Not all organisations have the budget for comprehensive ethical training.
- Complexity of AI Systems: Understanding how AI makes decisions can be difficult, making it hard to ensure ethics are followed.
Ethical AI design is not just about technology; it’s about creating systems that respect human values and promote fairness.
By focusing on these areas, we can work towards a future where AI is developed responsibly and ethically, ensuring it benefits everyone.
Human-Centric Approach to AI Development
Collaboration with Stakeholders
A human-centric AI system is built through collaboration among various stakeholders. This includes:
- Leaders who set the vision.
- UX designers who focus on user experience.
- Customer service representatives who understand user needs.
- Customers themselves, whose feedback is invaluable.
Involving users at every stage, from design to testing, ensures that the AI aligns with their expectations and requirements.
Transparency and Inclusivity
The decision-making process of AI should be clear and understandable. This helps build trust and ensures users feel in control. Key points include:
- Clear communication about how recommendations are made.
- Diverse perspectives to cater to a wide range of users, avoiding biases.
- User feedback mechanisms to adapt the AI system over time.
Continuous Learning and Improvement
AI systems should be designed to learn from user interactions. This allows them to:
- Adapt to changing user needs.
- Improve performance based on feedback.
- Evolve to meet new challenges.
A human-centric approach is essential for creating AI that truly serves people, rather than just optimising for efficiency.
By focusing on these principles, we can ensure that AI development prioritises human needs and values, leading to better outcomes for everyone.
AI’s Impact on Job Markets and Employment
Job Displacement and Creation
AI is changing the job landscape in various ways. AI can bring many benefits to the workplace such as higher productivity, improved job quality, and stronger occupational safety and health. However, it also poses challenges:
- Job Displacement: Many roles, especially those that involve repetitive tasks, are at risk of being automated.
- Creation of New Job Categories: New roles are emerging in AI development, management, and maintenance.
- Changing Skill Requirements: Workers need to adapt to new technologies and acquire different skills.
Strategies for Fair Transition
To ensure a smooth transition for workers affected by AI, several strategies can be implemented:
- Retraining Programmes: Offering training to help workers learn new skills.
- Social Safety Nets: Providing support for those who lose their jobs due to automation.
- Collaboration with Industries: Engaging with businesses to understand future job needs.
Human-AI Collaboration
AI should complement human skills rather than replace them. This collaboration can lead to:
- Enhanced productivity and efficiency.
- Improved job satisfaction as workers focus on more complex tasks.
- A more innovative work environment where human creativity is valued alongside AI capabilities.
The integration of AI into the workforce must be approached with care to ensure that it benefits everyone involved, not just a select few.
Ethical Implications of Generative AI
Benefits of Ethical AI Use
Using generative AI responsibly can lead to numerous advantages, such as:
- Building Trust: Ethical practises can enhance your reputation.
- Promoting Fairness: Generative AI can help create inclusive content.
- Encouraging Innovation: Responsible use can lead to new ideas and solutions.
Risks of Generative AI
While generative AI offers many benefits, it also poses certain risks:
- Hallucinations: AI may produce incorrect or misleading outputs.
- Deepfakes: Manipulated media can be used maliciously.
- Job Displacement: Automation may lead to job losses in some sectors.
Developing Risk Management Plans
To mitigate these risks, consider the following steps:
- Identify Use Cases: Clearly define how generative AI will be used in your organisation.
- Set Quality Standards: Establish criteria for evaluating AI outputs.
- Keep Humans Involved: Ensure that human oversight is maintained in decision-making processes.
The ethical use of generative AI is crucial for fostering a safe and innovative environment. By prioritising responsibility, we can harness its potential while minimising harm.
In summary, the ethical implications of generative AI are significant. By understanding both the benefits and risks, we can work towards a future where AI serves humanity positively. Empowering and reskilling the workforce is essential as we navigate this evolving landscape.
AI in Healthcare: Ethical Considerations
Patient Data Security
In the healthcare sector, protecting patient data is crucial. AI systems must ensure that sensitive information is handled securely to maintain trust. Here are some key points:
- Encryption: All patient data should be encrypted to prevent unauthorised access.
- Access Controls: Only authorised personnel should have access to sensitive information.
- Regular Audits: Conducting regular security audits helps identify vulnerabilities.
Decision-Making Support
AI can assist healthcare professionals by providing data-driven insights. This support is vital for accurate diagnoses and treatment plans. Important aspects include:
- Transparency: AI systems should explain their reasoning to ensure trust.
- Accuracy: Tools like PathAI help pathologists make precise diagnoses by analysing medical images.
- Collaboration: AI should complement human expertise, not replace it.
Transparency in Healthcare AI
Transparency is essential in AI applications within healthcare. It helps build trust and ensures ethical practises. Key considerations include:
- Clear Communication: Patients should understand how AI influences their care.
- Informed Consent: Patients must be aware of how their data is used.
- Accountability: There should be clear guidelines on who is responsible for AI decisions.
Ethical AI in healthcare is about ensuring health equity and addressing the risk of bias in algorithms. This is vital to prevent existing health disparities from worsening.
By focusing on these ethical considerations, we can harness the benefits of AI while safeguarding patient rights and well-being.
Building Public Trust in AI
Transparency in AI Operations
To build trust in AI, it is essential to ensure transparency in how AI systems operate. This means that users should understand how decisions are made. Here are some key points to consider:
- Clear Communication: Explain how AI works in simple terms.
- Open Data Practises: Share data sources and methods used in AI development.
- Regular Audits: Conduct audits to ensure fairness and accountability.
Accountability Measures
Accountability is crucial for fostering trust. Companies must take responsibility for their AI systems. This includes:
- Establishing Ethical Standards: Setting guidelines for AI development helps prevent harm and promotes beneficial outcomes.
- Legal Responsibility: Companies should be held accountable for any negative impacts caused by their AI systems.
- User Feedback: Encourage users to provide feedback on AI performance to improve systems continuously.
Ethical Data Use
Using data ethically is vital for maintaining public trust. Here are some practises:
- Data Protection Protocols: Implement strong measures to protect user data.
- Privacy-Enhancing Technologies: Use technologies that safeguard personal information.
- Transparency in Data Usage: Clearly inform users about how their data is used.
Building trust in AI requires a commitment to ethical standards and transparency. By prioritising these values, we can create AI systems that benefit everyone.
Balancing Innovation with Responsibility in AI
In the rapidly evolving world of artificial intelligence, it is crucial to find a balance between innovation and responsibility. This balance ensures that while we harness the potential of AI, we also safeguard against its risks.
Benefit Maximisation
- Focus on Public Good: AI should be developed to enhance societal welfare.
- Prioritise Positive Outcomes: Aim for advancements that benefit the majority.
- Engage with Communities: Involve various stakeholders to understand their needs and concerns.
Preventing Misuse and Harm
- Implement Security Measures: Protect AI systems from unauthorised access and misuse.
- Establish Clear Guidelines: Create rules for ethical AI use to prevent harmful applications.
- Regular Audits: Conduct assessments to ensure compliance with ethical standards.
Ensuring Equitable Treatment
- Avoid Bias: Strive for fairness in AI algorithms to ensure all demographics are treated equally.
- Inclusive Development: Involve diverse teams in AI design to consider various perspectives.
- Transparency in Processes: Maintain open communication about how AI systems operate and make decisions.
Balancing innovation with responsibility is not just a choice; it is a necessity for the sustainable development of AI technologies. By prioritising ethical considerations, we can foster trust and ensure that AI serves humanity positively.
This approach not only enhances the reputation of AI technologies but also encourages a culture of accountability and ethical responsibility among developers and users alike.
Addressing Bias and Discrimination in AI
Identifying Sources of Bias
Bias in AI can stem from several areas, which can lead to unfair outcomes. Here are the main sources:
- Biassed Training Data: AI systems learn from historical data, which may reflect existing societal biases. For example, an AI hiring tool developed by Amazon favoured male candidates because it was trained on resumes predominantly submitted by men.
- Algorithmic Bias: Flaws in algorithm design can introduce bias. In the criminal justice system, risk assessment tools have been found to unfairly predict re-offending rates based on race.
- Lack of Diversity in Development Teams: Homogeneous teams may overlook biases affecting underrepresented groups, leading to systems that do not serve everyone fairly.
Techniques to Mitigate Bias
To address these issues, several strategies can be employed:
- Diverse and Inclusive Data: Ensure training data represents various populations. For instance, Google has worked on creating inclusive datasets for facial recognition.
- Bias Detection Tools: Use tools like IBM’s AI Fairness 360 to identify and reduce bias in AI models.
- Regular Audits: Conduct audits of AI systems to maintain transparency and fairness in decision-making processes.
Case Studies of Bias in AI
Several real-world examples highlight the impact of bias in AI:
Case | Issue | Outcome |
---|---|---|
Amazon Hiring Tool | Gender bias due to training data | Discrimination against female candidates |
COMPAS Risk Assessment | Racial bias in predictions | Unfair treatment of Black defendants |
Facial Recognition Systems | Higher error rates for darker skin | Inaccurate identification in law enforcement |
Addressing bias in AI is not just a technical challenge; it is a moral imperative to ensure that technology serves all of society fairly. By prioritising fairness, we can create AI systems that uplift rather than marginalise.
Future Directions in AI Ethics
Emerging Ethical Challenges
As AI technology continues to evolve, new ethical challenges arise. Key areas to watch include:
- Data Privacy: Ensuring that personal information is protected as AI systems become more integrated into daily life.
- Bias in Algorithms: Addressing the potential for AI to perpetuate or even amplify existing biases in society.
- Autonomous Decision-Making: Evaluating the implications of AI systems making decisions without human intervention.
Innovations in Ethical AI
The future of AI ethics will likely see significant innovations aimed at promoting responsible use. Some promising developments include:
- Ethical AI Frameworks: Establishing guidelines that ensure AI systems are designed with ethical considerations in mind.
- Collaborative Governance: Involving diverse stakeholders in the decision-making process to enhance accountability.
- AI Auditing Tools: Creating tools that can assess AI systems for ethical compliance and bias detection.
Global Standards and Regulations
To ensure responsible AI development, there is a growing need for global standards and regulations. This includes:
- International Cooperation: Countries working together to create a unified approach to AI ethics.
- Regulatory Bodies: Establishing organisations that oversee AI development and enforce ethical standards.
- Public Engagement: Encouraging community involvement in discussions about AI ethics to foster transparency and trust.
The future of AI ethics is not just about preventing harm; it’s about actively promoting the benefits of ethical AI use for society as a whole.
In conclusion, as AI continues to advance, it is crucial to remain vigilant and proactive in addressing ethical concerns, ensuring that innovation does not come at the cost of responsibility.
Conclusion
In summary, when harnessed properly, AI can be a powerful ally rather than a threat. It is essential for us, as leaders and developers, to strike a careful balance in its use. By setting clear rules and ethical guidelines for AI development and application, we can ensure that the safety and well-being of individuals take precedence over mere technological advancement or profit. This approach not only fosters trust but also promotes a future where AI serves humanity positively.
Frequently Asked Questions
What are the main principles of AI ethics?
The key principles include fairness, transparency, accountability, and privacy. These principles aim to ensure that AI systems are fair, open, responsible, and respect people’s privacy.
Why is human oversight important in AI systems?
Human oversight is crucial because it helps prevent mistakes and ensures that AI systems work as intended. Humans can monitor AI actions and make necessary adjustments.
How can we protect data privacy when using AI?
To protect data privacy, we should implement strict security measures, use privacy-enhancing technologies, and have clear data access policies.
What does ethical AI design involve?
Ethical AI design involves embedding ethical considerations into the development process, ensuring that AI systems are built with fairness and accountability in mind.
How can we build trust in AI?
Building trust in AI can be achieved by being transparent about how AI systems work, ensuring accountability, and using data responsibly.
What are the risks of generative AI?
Generative AI can create misleading or harmful content. It’s important to understand these risks and develop strategies to manage them.
How does AI affect jobs?
AI can lead to job displacement but also creates new job opportunities. It’s important to prepare workers for these changes through reskilling and support.
What are the future challenges in AI ethics?
Future challenges include addressing emerging biases, ensuring equitable access to AI technologies, and developing global regulations to govern AI use.
-
Home and Garden4 days ago
Transform Your Space: A Guide to Minimalist House Interior Design in 2025
-
Innovations6 days ago
Will AI Take Your Job? The Truth About Automation and Employment
-
Home & Family4 days ago
10 Essential Cleaning Hacks for Bathroom Surfaces You Need to Try
-
Crime4 days ago
Exploring the Most Anticipated New True Crime Documentaries of 2025
-
Health & Fitness4 days ago
Discovering the Best Multivitamin for Women Over 40: Essential Nutrients for Optimal Health
-
Business4 days ago
Maximize Your Earnings with American Express High Yield Savings Accounts in 2025
-
Health & Fitness4 days ago
Discover the Best Multivitamin for Women Over 40: A Comprehensive Guide to Optimal Health
-
Artificial Intelligence4 days ago
Breaking Generative AI News: Innovations Shaping the Future of Technology